Disclosure-centered mediation
TwSw interventions are centered on the agency and autonomy of individuals in their repeated interactions with AI models. We propose the use of disclosures of the potential for algorithmic harms ex-ante as well as the use of disclosures to facilitate a mediation process when harms occur.
Mediation practices, including apology, have played a significant role in existing fields of law, particularly in circumstances of medical error. Expressions of regret recognize imperfection and the space for change. Similarly, we see TwSw as embodying an analogous enforcement mechanism; one that can enable an alternative apology-centered mediation.
We encourage practitioners to ask: What does meaningful consent mean? How would you expand traditional terms of service, privacy policies, community guidelines, and other end-user license agreements to include disclosure about the use of AI and its potential impacts? What needs to be disclosed and to whom? How could we enable safe collective sensemaking with regards to potential harms due to protected class attributes (i.e. gender, race) used or inferred by the AI systems? What actions can be taken as part of a disclosure-centered transformative justice approach to mediation of algorithmic harms or risks?
Resources:
- Cohen IG (2019) Informed consent and medical artificial intelligence: What to tell the patient? The Georgetown Law Journal 108: 1425-1469.
- Costanza-Chock S, Raji ID and Buolamwini J (2022) Who Audits the Auditors? Recommendations from a field scan of the algorithmic auditing ecosystem. In Proceedings of the 2022 ACM Conference on Fairness, Accountability, and Transparency (pp. 1571-1583).
- Chipidza FE, Wallwork RS and Stern TA (2015) Impact of the doctor-patient relationship. The Primary Care Companion for CNS Disorders 17(5): 27354.
- Davis JL, Williams A, and Yang MW (2021) Algorithmic reparation. Big Data & Society 8(2).
- Ho DE (2012) Fudging the nudge: Information disclosure and restaurant grading. The Yale Law Journal 122: 574-688.
- Raji ID, Kumar IE, Horowitz A and Selbst A (2022) The fallacy of AI functionality. In Proceedings of 2022 ACM Conference on Fairness, Accountability, and Transparency (pp. 959-972)
- Norval C, Cornelius K, Cobbe J and Singh J (2022) Disclosure by design: Designing information disclosures to support meaningful transparency and accountability. In Proceedings of the 2022 ACM Conference on Fairness, Accountability, and Transparency (pp. 679-690)
- Robbennolt JK (2009) Apologies and medical error. Clinical Orthopaedics and Related Research 467(2): 376-382
- Wall JA and Dunne TC (2012) Mediation research: A current review. Negotiation Journal, 28(2), pp.217-244
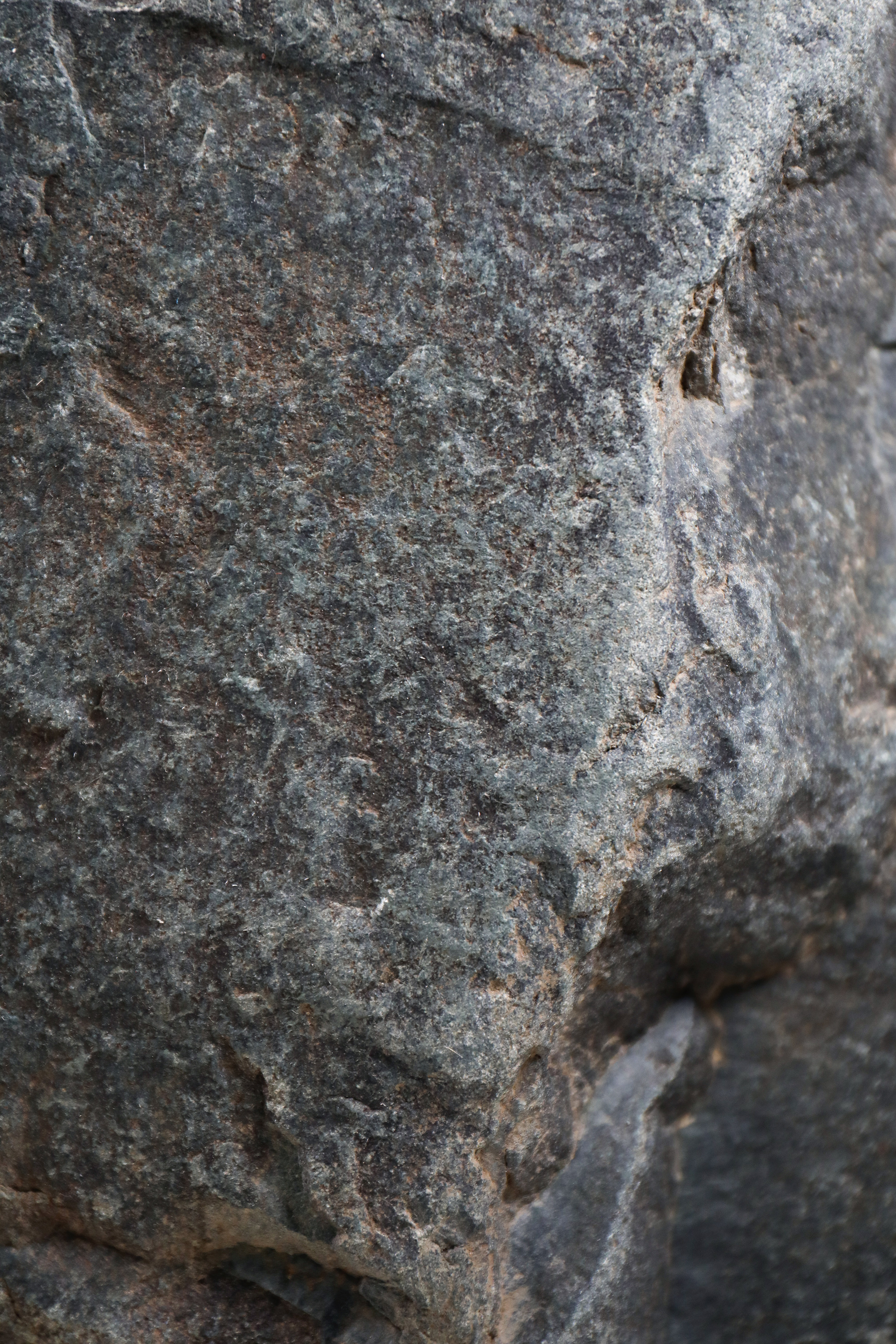
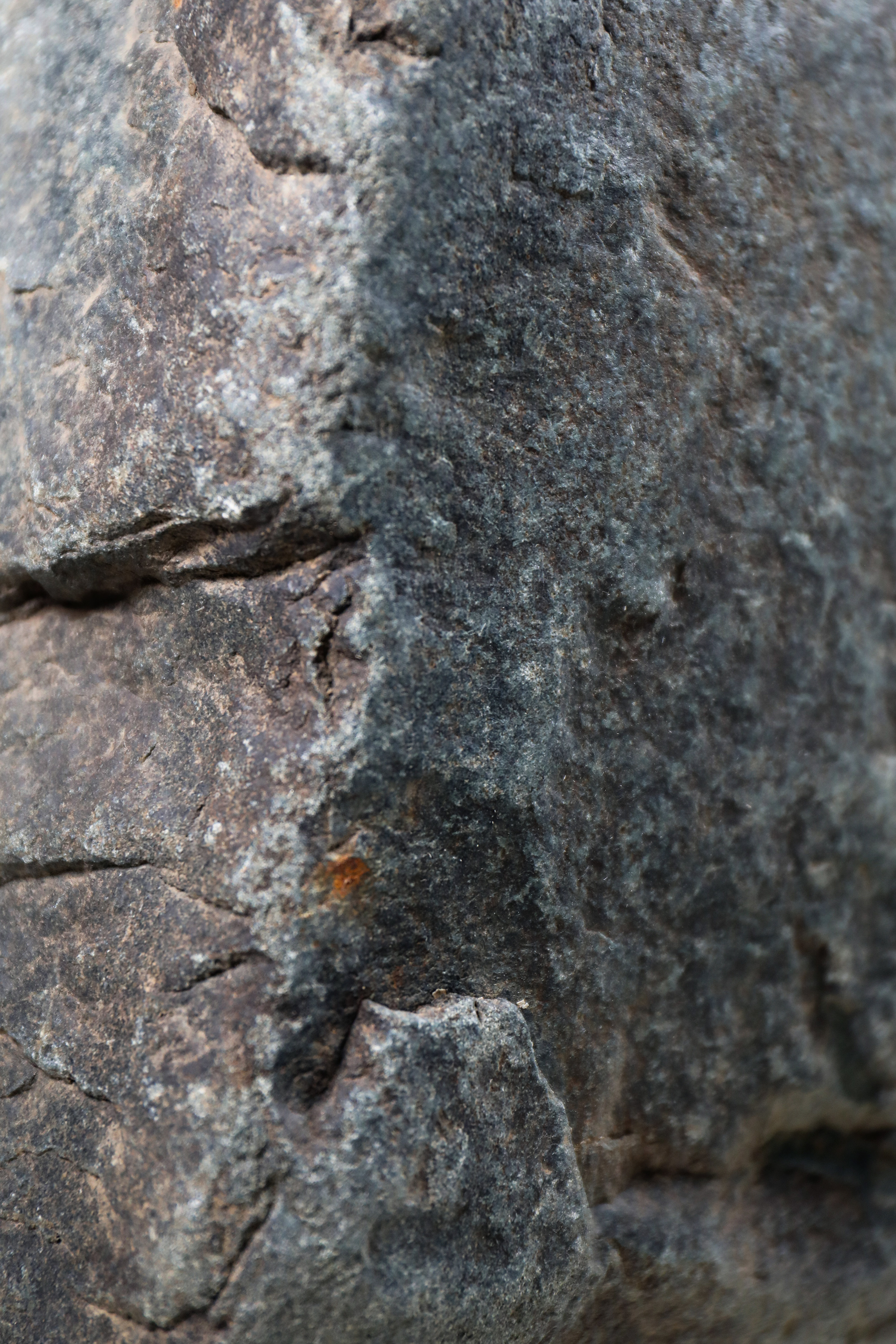
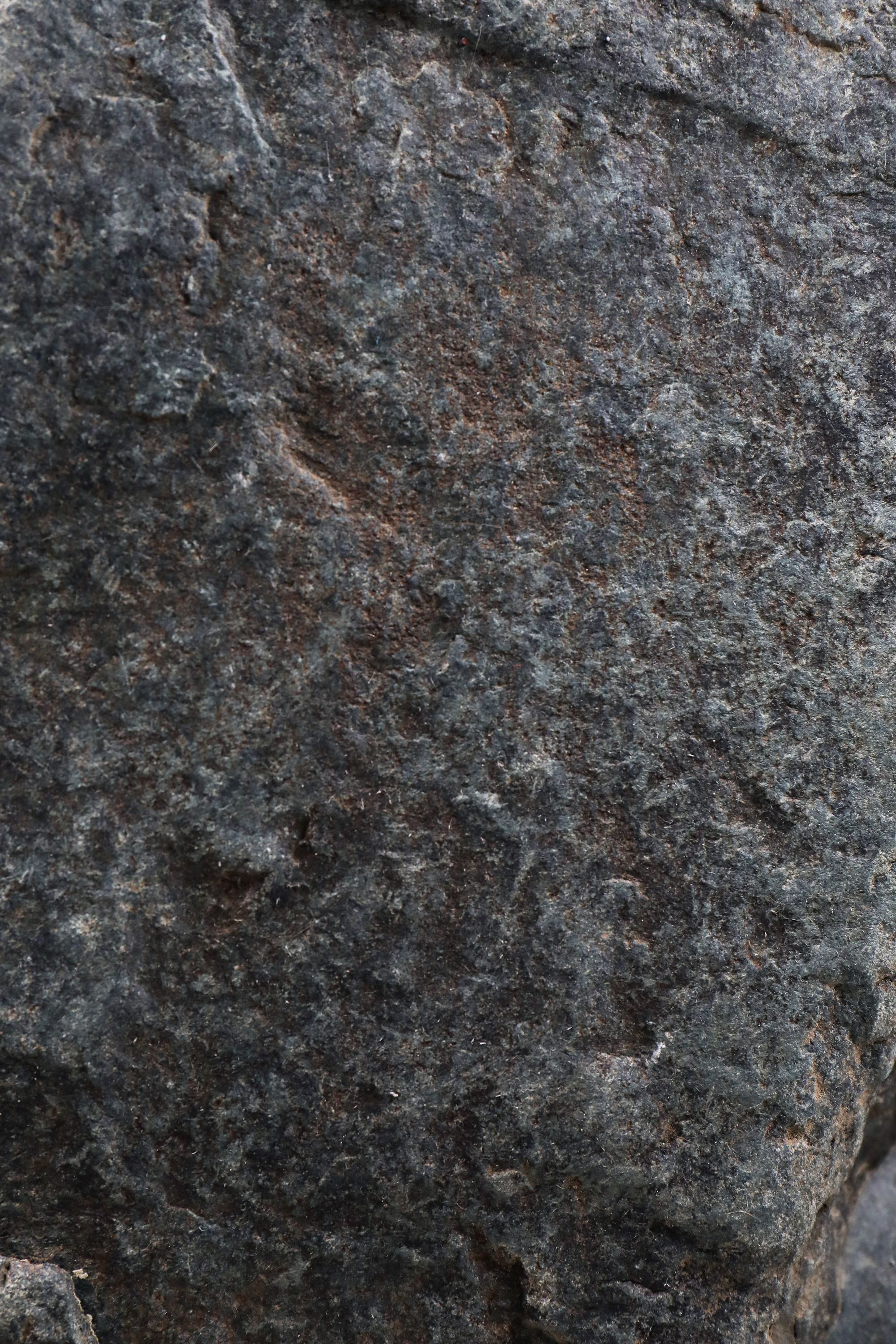
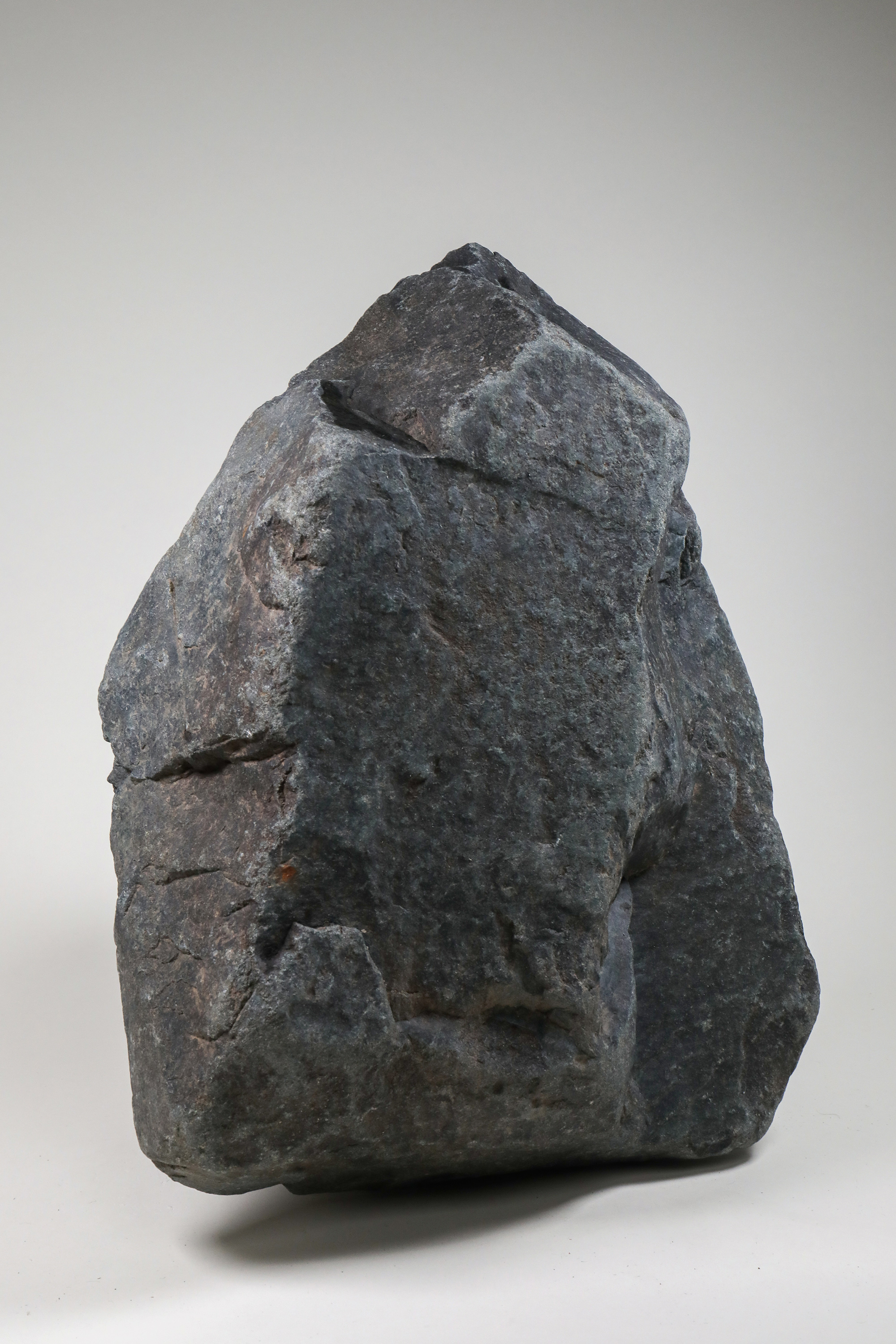